Comparator-Based Methods for Quantifying Antitrust Damages
One of the most widely used approaches for estimating antitrust damages involves comparator-based methods, which rely on comparing the actual scenario to a counterfactual—what would have happened if the infringement hadn't occurred.
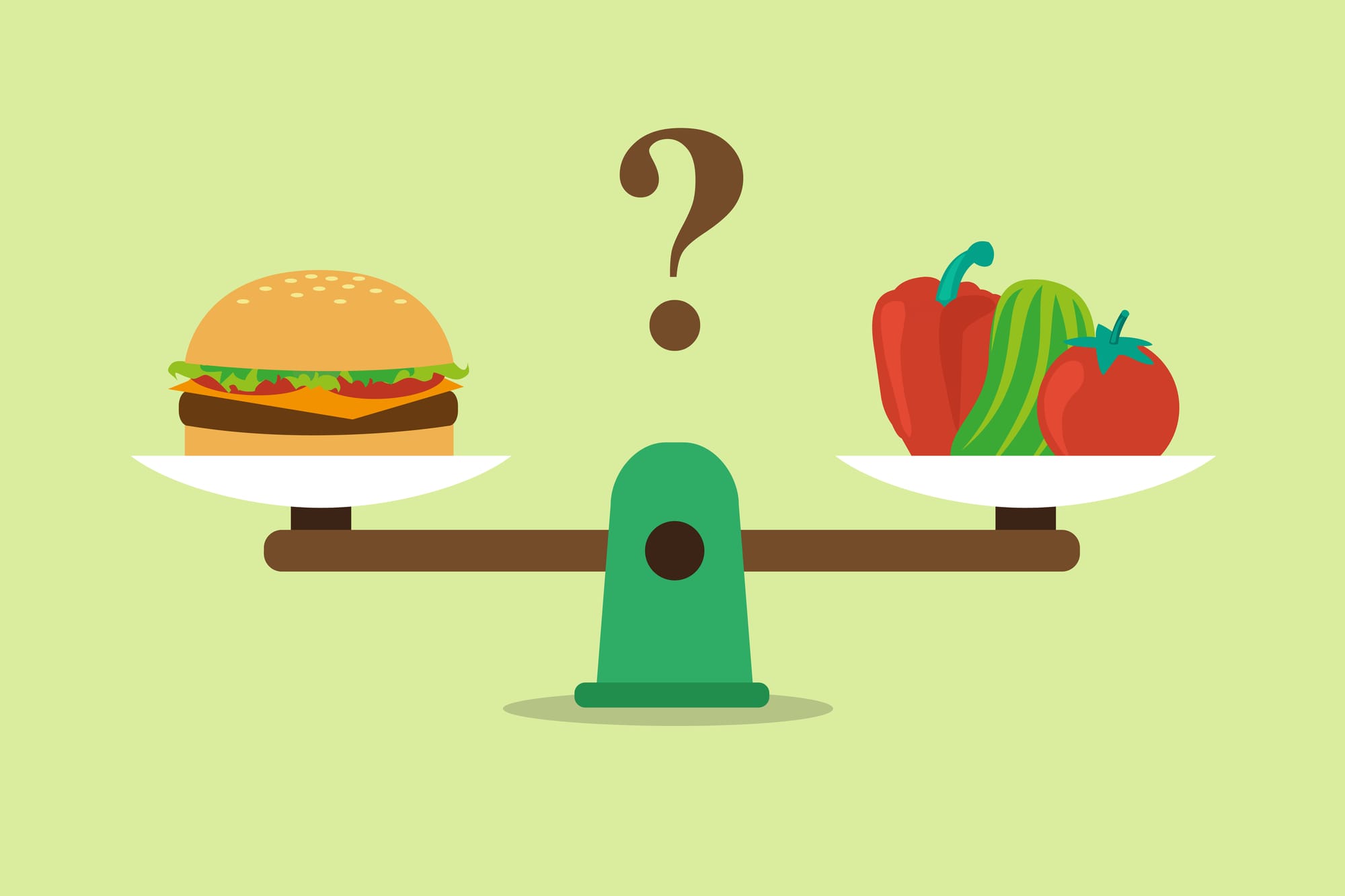
Estimating damages in antitrust cases can be complex, requiring robust methodologies to ensure accurate and fair outcomes. We discussed a highly summarized overview of the various considerations in estimating antitrust damages in our article on Overview of Antitrust Damages Estimation. One of the most widely used approaches involves comparator-based methods, which rely on comparing the actual scenario to a counterfactual—what would have happened if the infringement hadn't occurred. In essence, these methods establish a "but-for" scenario to determine how prices, profits, or market shares would have behaved in the absence of anti-competitive practices like cartels or monopolistic behavior.
In this article, we'll break down various comparator-based techniques, explore their strengths and weaknesses, and provide practical examples to illustrate how they work. Going into the complexities of each of these methods can take pages on their own, so we will focus on giving you a brief introduction to these methods for now. Future articles will focus on some of these methods individually giving you a better sense of each of them.
What Are Comparator-Based Methods?
Comparator-based methods leverage data from comparable markets, periods, or firms to estimate the "but-for" scenario. They are commonly used in antitrust cases to calculate damages by estimating overcharges or lost profits due to anti-competitive conduct. The key to these methods is identifying a suitable comparator—a market, period, or dataset unaffected by the infringement, which serves as the benchmark for comparison.
Why Are Comparators Useful?
By using comparable groups or time periods, these methods aim to isolate the impact of the infringement on prices or other market outcomes. They also help address the challenge of determining what would have occurred if the anti-competitive actions had not taken place, thereby providing a basis for calculating damages.
Common Applications:
- Estimating overcharges in price-fixing or cartel cases.
- Assessing the impact of exclusionary practices on market entry.
- Quantifying lost profits due to monopolistic behaviors.
1. Comparison of Averages: A Simple Approach
One of the most straightforward comparator-based techniques is the comparison of averages. This method involves comparing the average price in an unaffected market or period to the price in the affected market.
How it Works
Suppose there are five unaffected markets with an average price of €10. If the price in the cartelized market is €12, the overcharge can be estimated as €2 (a 16.7% increase). The difference represents the estimated damage due to the anti-competitive behavior.
Benefits and Limitations
- Benefits: Simple to understand and apply, requires minimal data, and provides quick estimates.
- Limitations: This method assumes that the comparator market is truly unaffected by the infringement and that no other factors are influencing price differences. It's crucial to validate findings with statistical tests to ensure robustness.
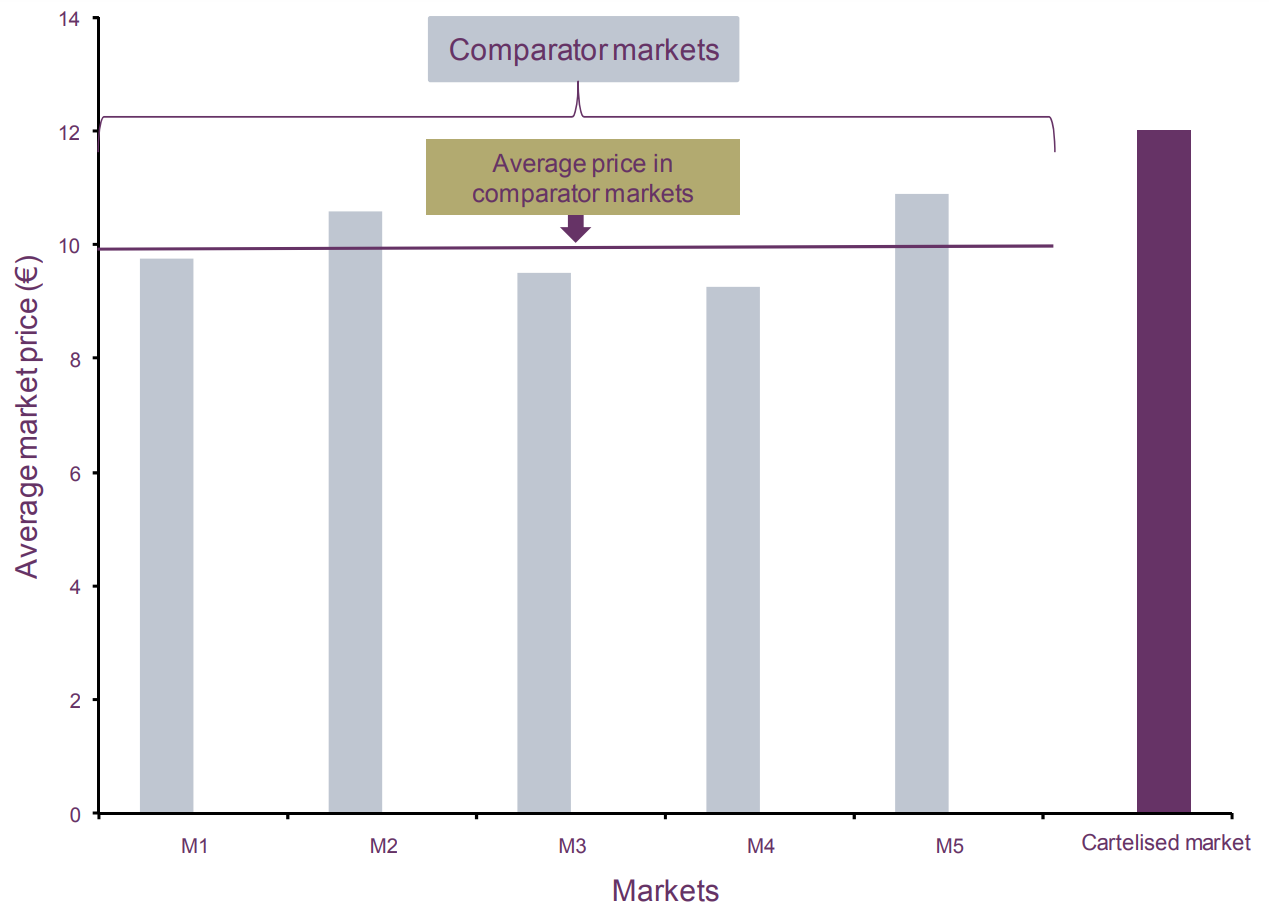
2. Interpolation: Bridging Gaps in Data
Interpolation builds on the comparison of averages by considering prices before and after the infringement period to estimate what prices would have been during the infringement.
How It Works
Assume we have data on prices before a cartel formed (say €8) and after it ended (say €9). Interpolation estimates the prices that would have prevailed during the cartel period based on the trend from the surrounding periods. By "joining the dots" between pre- and post-infringement prices, this method provides a counterfactual estimate of prices had the cartel not existed.
When to Use
This approach is ideal when reliable data is available for periods surrounding the infringement but not during the infringement itself. However, it assumes that the factors influencing prices before and after the infringement would have continued in a similar fashion during the infringement period.
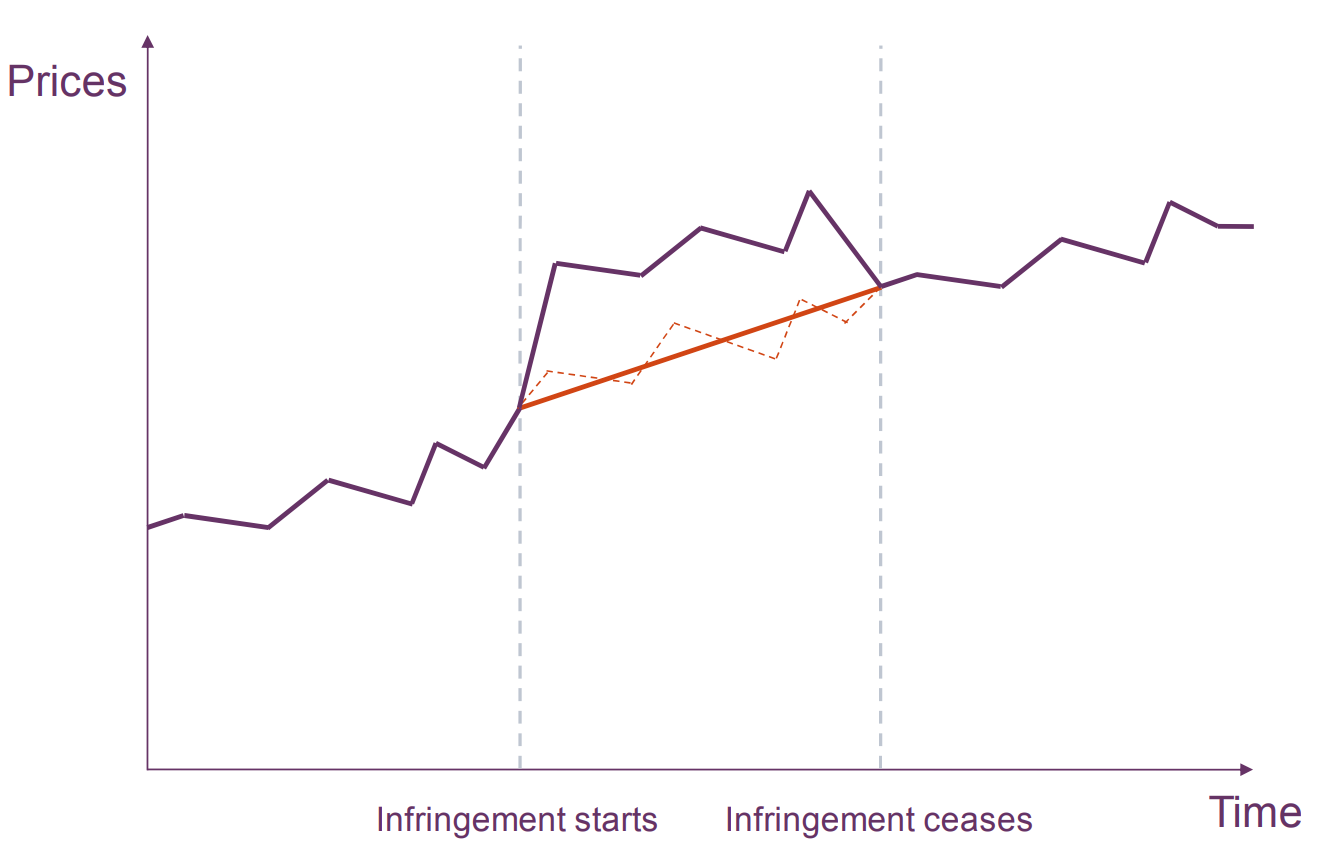
3. Regression Techniques: A More Nuanced Approach
While simple comparisons are quick, they often overlook other factors influencing prices. Regression analysis is a more sophisticated tool that controls for multiple variables to better isolate the effect of the infringement.
How It Works
In a regression model, the dependent variable (e.g., price) is explained using various independent variables such as:
- Input costs (e.g., raw materials, labor).
- Market characteristics (e.g., product quality, competition intensity).
- Time trends or seasonal effects.
By controlling for these factors, regressions help isolate the impact of the anti-competitive behavior itself. For example, a regression might reveal that input costs rose by 5% during the cartel period, but prices increased by 20%, suggesting a significant overcharge due to the cartel.
Benefits
- Flexibility: Can incorporate multiple variables to account for complex market dynamics.
- Precision: Provides statistically robust estimates by controlling for confounding factors.
Challenges
- Requires large and high-quality datasets.
- May involve complex statistical analysis, making it less accessible to non-experts.
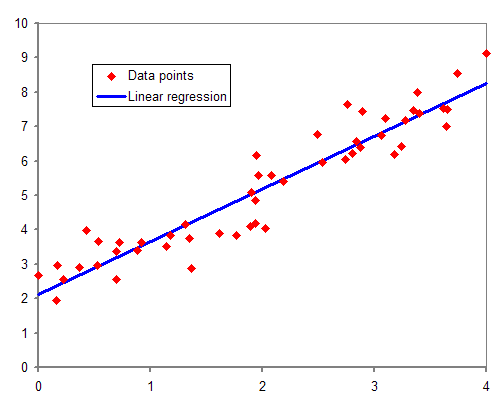
4. Cross-Sectional vs. Time-Series Comparisons
Comparator-based methods can also vary based on whether the comparison is across markets (cross-sectional) or over time (time-series).
Cross-Sectional Comparisons
- Compare prices between affected and unaffected markets at the same point in time.
- Ideal when there's uncertainty about the timing of the infringement.
- Assumes that comparator markets are sufficiently similar to the affected market.
Time-Series Comparisons
- Analyze prices within the same market across different time periods (before, during, and after the infringement).
- Provides a like-for-like comparison since it uses the same market, but requires precise knowledge of when the infringement started and ended.
- Can be influenced by external factors that change over time (e.g., macroeconomic conditions).
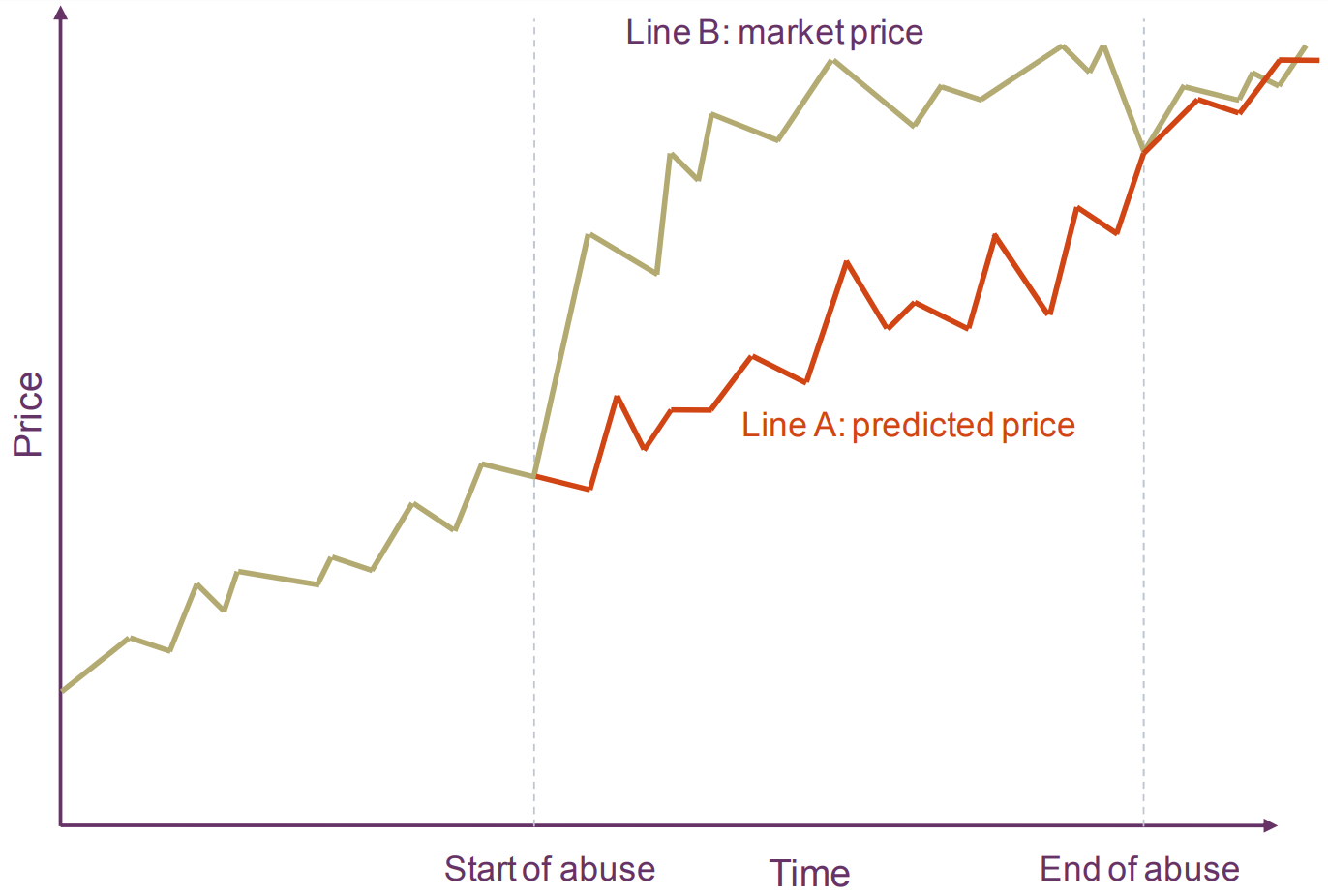
5. Difference-in-Differences (DiD): Controlling for External Factors
A more advanced technique is the Difference-in-Differences (DiD) approach, which addresses the limitations of both cross-sectional and time-series methods.
How It Works
The DiD method compares the changes in prices over time between an affected market (with infringement) and an unaffected comparator market (without infringement). This technique controls for external factors by examining how prices in both markets evolve over time.
For instance, if prices in both markets were rising due to inflation, the DiD approach would adjust for this common trend, attributing only the remaining difference to the infringement.
Benefits
- Robustness: Controls for time trends that may affect both the treated (affected) and control (unaffected) groups.
- Versatility: Useful in situations where the timing of the infringement is uncertain or where external factors are influencing both markets.
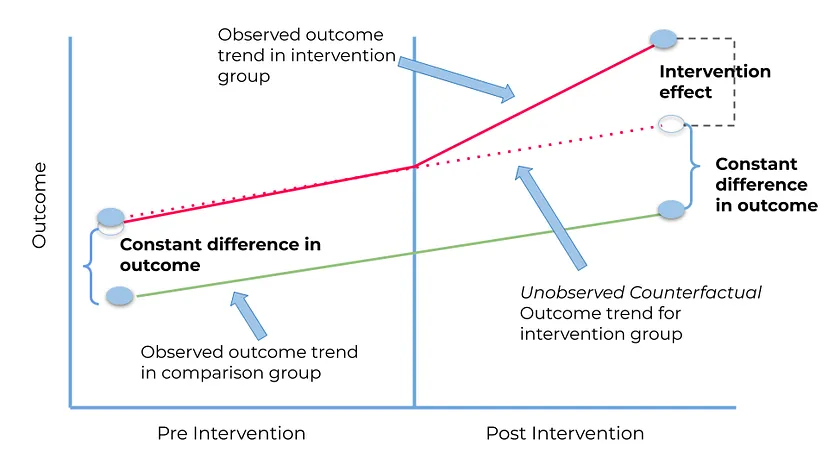
Choosing the Right Method
Each comparator-based method has its strengths and is suited to different types of data and market conditions. While simpler techniques like averages and interpolation are easier to apply, they may not account for confounding variables. More sophisticated methods like regression and Difference-in-Differences provide more accurate estimates but require deeper statistical expertise and richer data.
The choice of method depends on factors such as data availability, the complexity of the market, and the nature of the infringement. By selecting the appropriate technique, experts can ensure more accurate damage estimations, leading to fairer outcomes in antitrust litigation.